PyTorch torch.split() function splits the tensor into equal-sized or custom-sized chunks (multiple sub-tensors) along a specified dimension. It returns a tuple of tensors. You have to loop through a tuple to get the batch one by one.
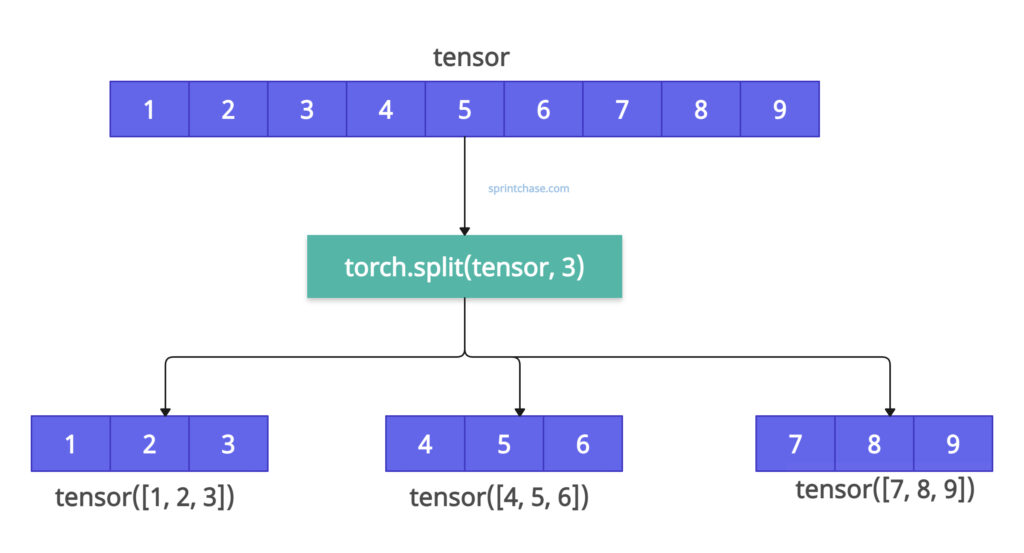
Syntax
torch.split( tensor: Tensor, split_size_or_sections: int | Sequence[int], dim: int = 0 ) → Tuple[Tensor, ...]
Parameters
Name | Value |
tensor | It is an input tensor that needs to be splitted. |
split_size_or_sections | It is the size of the chunk. It can be an integer or a list. |
dim | It is the axis (dimension) along which to split. By default, it is 0. |
Splitting a tensor into mini-batches
import torch tensor = torch.arange(1, 10) splitted_tensor = torch.split(tensor, 3) print(splitted_tensor) # Output: (tensor([1, 2, 3]), tensor([4, 5, 6]), tensor([7, 8, 9])) for i, batch in enumerate(splitted_tensor): print(f"Batch {i}: {batch}") # Output: # Batch 0: tensor([1, 2, 3]) # Batch 1: tensor([4, 5, 6]) # Batch 2: tensor([7, 8, 9])
We used the arange() function to create a 1D tensor of 9 elements. We divided the tensor into batches of 3. And using a for loop and enumerate(), we printed one by one, in batches, in the console. You can see that we passed the size 3, which is an integer.
Splitting by custom section sizes
Let’s create a random tensor from a normal distribution using torch.randn() function and split it by a list of sections along with columns.
import torch tensor = torch.randn(4, 10) sections = [3, 3, 4] t1, t2, t3 = torch.split(tensor, sections, dim=1) print(t1.shape, t2.shape, t3.shape) # Output: torch.Size([4, 3]) torch.Size([4, 3]) torch.Size([4, 4])
By passing dim = 1, we split a tensor along its columns. The [3, 3, 4] list specifies how many columns we need in each split. The numbers must sum to the size of the chosen dimension—in this case, 3 + 3 + 4 = 10.
Zero-length split
You cannot split a tensor with an empty list ([]) size because the sections list must sum to the dimension size.
If you try to execute this, it will throw RuntimeError.
import torch tensor = torch.randn(4, 10) empty_section = [] t0 = torch.split(tensor, empty_section, dim=1) print(t0) # Output: RuntimeError: split_with_sizes expects split_sizes to sum exactly to 10 (input tensor's size at dimension 1), # but got split_sizes=[]
Splitting an empty tensor
If you split an empty tensor, it returns an empty tuple. To create an empty tensor, we have used torch.empty() method.import torch # Creating an empty 1-D tensor (size 0) tensor = torch.empty(0) # Define an empty list of split sizes empty_sections = [] # Attempt to split the tensor into sections if empty_sections: parts = torch.split(tensor, empty_sections, dim=0) else: # no sections → no splits → empty tuple parts = () print(parts) # Output () print(type(parts)) # Output: <class 'tuple'>
You can see that since the tensor is empty, the output tuple is empty, and we verified that its class is a tuple.